Involvement in large-scale programs
DFG Priority Program Nano Security (Coordination) / DFG Schwerpunktprogramm Nano Security (Role: Coordination)
Started in 2022
Partners: 23 Primary Investigators from 17 Universities and research institutes in Germany
Summary: Today’s societies critically depend on electronic systems. Past spectacular cyber-attacks have clearly demonstrated the vulnerability of existing systems and the need to prevent such attacks in the future. The majority of available cyber-defenses concentrate on protecting the software part of electronic systems or their communication interfaces. However, manufacturing technology advancements and the increasing hardware complexity provide a large number of challenges so that the focus of attackers has shifted towards the hardware level. We are facing completely new security challenges due to the ongoing transition to radically new types of nano-electronic devices, such as memristors, spintronics, or carbon nanotubes. The main objective of the Priority Program Nano Security is to understand the implications of emerging nano-electronics to system security. Specifically, it assesses possible security threats and vulnerabilities stemming from novel nano-electronics – due to fundamental properties of nano-electronic devices, or to designers neglecting security – taking security into account during the complete development and product life cycle. It also develops innovative approaches for system security based on nano-electronics, including hardware trust anchors, which are hard to design with current technologies.
Representative publication
2021
- Nano Security: From Nano-Electronics to Secure Systems. Ilia Polian; Frank Altmann; Tolga Arul; Christian Boit; Ralf Brederlow; Lucas Davi; Rolf Drechsler; Nan Du; Thomas Eisenbarth; Tim Güneysu; Sascha Hermann; Matthias Hiller; Rainer Leupers; Farhad Merchant; Thomas Mussenbrock; Stefan Katzenbeisser; Akash Kumar; Wolfgang Kunz; Thomas Mikolajick; Vivek Pachauri; Jean-Pierre Seifert; Frank Sill Torres and Jens Trommer. In Proceedings of the Conference on Design, Automation & Test in Europe (DATE′21), Virtual Event, 2021.
Graduate School Intelligent Methods for Test and Reliability (Role: Director)
Started in 2019
Partners: Advantest Europe and 8 institutes of University of Stuttgart [hier Link zu https://www.gs-imtr.uni-stuttgart.de/team/principal_investigators/ ]
Summary: Today's economy and entire society rest upon the dependability of information technology and especially of the underlying hardware infrastructure. The "Internet of Things" affects nearly all aspects of human life, introduces severe vulnerabilities into the society and relies on strong actions towards reliability, safety, and security of information technology. Examples are autonomous systems including drones and self-driving cars or infrastructure networks including communication and energy. Thoroughly tested systems are mandatory for a responsible use of technology. The Graduate School Intelligent Methods for Test and Reliability (GS-IMTR) at the University of Stuttgart is funded by Advantest, one of the world leaders in Automated Test Equipment. Around ten PhD candidates and one Junior Professor will work together towards new solutions. The Graduate School’s scope includes topics such as design for test and diagnosis; post-silicon validation; test generation and optimization; robust device tuning; system-level test; lifetime test and reliability management; and test automation. A modern understanding of these topics demands novel artificial intelligence methods and has tight connections to data science, data analytics, data understanding, visualization, security, and privacy.
Representative publication
2022
- Intelligent Methods for Test and Reliability. Hussam Amrouch; Jens Anders; Steffen Becker; Maik Betka; Gerd Bleher; Peter Domanski; Nourhan Elhamawy; Thomas Ertl; Athanasios Gatzastras; Paul R. Genssler; Sebastian Hasler; Martin Heinrich; André van Hoorn; Hanieh Jafarzadeh; Ingmar Kallfass; Florian Klemme; Steffen Koch; Ralf Küsters; Andrés Lalama; Raphael Latty; Yiwen Liao; Natalia Lylina; Zahra Paria Najafi-Haghi; Dirk Pflüger; Ilia Polian; Jochen Rivoir; Matthias Sauer; Denis Schwachhofer; Steffen Templin; Christian Volmer; Stefan Wagner; Daniel Weiskopf; Hans-Joachim Wunderlich; Bin Yang and Martin Zimmermann. In Design, Automation & Test in Europe Conference & Exhibition, DATE 2022, 2022, pp. 1–6.
Ongoing Projects
Quantum computing engineering for multi-QPU architectures (IQST Graduate School @QuantumBW)
Started in 2025
Partner: Dr. Sebastian Brandhofer, IBM Böblingen
Supported groupmember: N.N.
Summary: Practical quantum advantage can only be realized on systems with a large number of qubits, and first quantum processing units (QPUs) with more than 1,000 qubits have emerged. For most hardware modalities, large-scale quantum computers will require a modular approach where units on different hierarchy levels are connected by either classical or quantum links. We distinguish between “islands of qubits” within a QPU, QPUs within one multi-QPU module, and several such modules that constitute what we call a multi-QPU architecture. This project aims at development of quantum computer engineering for multi-QPU architectures, connecting quatum algorithms and quantum hardware, encompassing tasks such as transpilation or error mitigation. Such tasks are heavily impacted by both the relatively large number of qubits and their heterogeneous connections by regular links and new inter- and intra-QPU couplers, and approaches for them in the multi-QPU context are lacking so far. We will also tackle entirely new multi-QPU problems, such as task migration between QPUs, multi-level transpilation with more than 2 hierarchy levels, and allocation of ancilla qubits to QPUs. This will necessitate new formalisms to represent QPUs with various quantum and classical couplers acting over short, medium and long range. With the involvement of IBM Quantum in Böblingen, we have access to a world leader in multi-QPU systems located 14 minutes away from our lab.
SeMSiNN: Secure Mixed-SIgnal Neural Networks (Project in DFG Priority Program Nano Security)
Started in 2024
Partner: Prof. Maurits Ortmanns, University of Ulm
Supported groupmember: Devanshi Upadhyaya
Summary: Artificial intelligence (AI) has revolutionized our lives. Edge AI, with AI workloads running on resource-restricted “edge devices” with limited or no communication with a remote server offers a number of advantages: it saves transmission power; avoids communication delays; eliminates possible reliability issues; and evades security vulnerabilities due to eavesdropping or manipulation of the transmitted data. At the same time, the transition to Edge AI shifts the overall system’s attack surface, because edge devices are usually designed to be exposed to their users, thus giving potential attackers physical access to components on which sensitive data are stored and processed. To reduce the energy demands of edge AI, one promising line of research uses mixed-signal (MS) circuits nased on analog quantities, such as current and charge. This project aims to methodically approach the security of quantized and pruned convolutional neural-networks (CNNs) for power-constrained MS edge applications by providing suitable models, simulation tools, and network improvements on all hierarchy levels to optimize not only considering cost vs. accuracy but also including security.
Representative publications
2024
- Enabling Power Side-Channel Attack Simulation on Mixed-Signal Neural Network Accelerators. Simon Wilhelmstätter; Joschua Conrad; Devanshi Upadhyaya; Ilia Polian and Maurits Ortmanns. In 2024 IEEE International Conference on Omni-layer Intelligent Systems (COINS), 2024, pp. 1–5. DOI: https://doi.org/10.1109/COINS61597.2024.10622156
- Attacking a Joint Protection Scheme for Deep Neural Network Hardware Accelerators and Models. Simon Wilhelmstätter; Joschua Conrad; Devanshi Upadhyaya; Ilia Polian and Maurits Ortmanns. In 2024 IEEE 6th International Conference on AI Circuits and Systems (AICAS), 2024, pp. 144–148. DOI: https://doi.org/10.1109/AICAS59952.2024.10595935
Quantum Algorithm Compilation for Rydberg Quantum Computers (IQST Graduate School Project)
Started in 2023
Partner: Hans-Peter Büchler, Institute for Theoretical Physics III, University of Stuttgart
Supported groupmember: Biswash Ghimire
Summary: Within the last few years, the goal to build a quantum computer based on neutral atoms has seen some tremendous breakthroughs. Rydberg platforms combine high-fidelity two-qubit quantum gates and natural long coherence times with the possibility to scale the number of qubits up to 500. This project aims at developing and analysing methods for quantum algorithm compilation for the Rydberg platform. Quantum algorithm compilation takes a quantum algorithm and translates it into instructions that can be executed on a given quantum computer, optimizing parameters such as the duration and/or the fidelity of the execution. The Rydberg platform provides a number of unusual features that can benefit compilation: the availability of 𝑛-qubit gates and the option of changing the qubit connectivity on-the-fly by “Rydberg shifts”. The goal of this project is to provide an integrated compilation approach that will also incorporate the deep knowledge about the optimal control pulses for the Rydberg platform to optimize entire circuits.
Enhancing Test Methods by Magnetic Fields (GS-IMTR Project P12)
Started in 2020
Partners: Prof. Hussam Amrouch (TU Munich), Prof. Jens Anders, Institute of Smart Sensors, University of Stuttgart
Supported groupmember: Karthik Pandaram
Summary: Integrated circuits for quality-sensitive applications, such as automotive, aerospace, medical and production systems, require an improved coverage of manufacturing defects, including hidden latent defects. To improve the detection of such defects, it has been suggested to apply tests under non-nominal conditions. A number of stress test methods (e.g., elevated-voltage, elevated-temperature testing) and screening methods (e.g., low-voltage testing) have been evaluated theoretically and experimentally. This project opens a new dimension in IC quality assurance: enhancing test application by controlled magnetic fields, enabled by HProbe's IBEX test equipment. We expect threefold potential improvements: First, better coverage of parametric defects, which introduce or modify transistor characteristics (e.g., channel conductance), parasitic resistances, capacitances or inductances within the circuit, rather than disrupting it completely leading to hard fails. Second, exposure of early-life failures that did not, at the time of test application directly after manufacturing, develop a sufficiently strong impact to be detectable directly. Third, assistance during failure analysis, where a defective circuit location might produce a distinct response, compared to its other parts, when a magnetic field is present.
Representative publication
2024
- Optimized detection of marginal defects in standard cells using unsupervised learning. Karthik Pandaram; Hussam Amrouch and Ilia Polian. In 2024 Proc. of IEEE Asian Test Symp. (ATS), 2024, pp. 1–6. DOI: https://doi.org/10.1109/ATS64447.2024.10915423
AI-DeTeC: AI-Driven Device-specific Tester Calibration: Concept, Development, Monitoring (funded by Infineon Technologies and Advantest)
Started in 2022
Supported groupmember: Anand Venkatachalam
Summary: This project aims at improving key test performance properties by correlating data from the device under test and from the test cell (automatic test equipment, loadboard, pogo, socket), using advanced data-analytics techniques. Artificial intelligence-based methodologies for analysis of data from various sources during test will be established, with the purpose to improve both: the quality of the product (yield) and the efficiency of the test process itself (overall equipment effectiveness). Specifically, we will create a high-level predictive AI model to bring together test-related data from the device under test (both its regular responses and acquisition of on-chip parameters); the loadboard measurements; the tester-internal equipment data; the history of maintenance events; and yield-related information. This model should be able to predict relevant yield detractors and automated test equipment related variables such as calibration intervals.
Representative publications
2025
- Intelligent Device Tester Calibration Based on Gauge Repeatability and Reproducibility. Anand Venkatachalam; Ernst Aderholz; Matthias Sauer; Simon Schweizer; Matthias Werner and Ilia Polian. In Proceedings of the 37th ITG / GMM / GI -Workshop Testmethoden und Zuverlässigkeit von Schaltungen und Systemen, Berlin, DE, 2025.
- Automated test equipment drift characterization based on gauge repeatability and reproducibility. A Venkatachalam; E Aderholz; M Sauer; S Schweizer; M Werner and I Polian. In IEEE European Test Symp. (ETS), Tallinn, EE, 2025.
MemCrypto: Towards Secure Electroforming-free Memristive Cryptographic Implementations (Project in DFG Priority Program Nano Security)
Started in 2021
Partner: Dr. Nan Du, Friedrich-Schiller University of Jena
Supported groupmember: Li-Wei Chen
Summary: Memristive devices offer enormous advantages for non-volatile memories and neuromorphic computing, but there is a rising interest in using memristive technologies for security applications. Project MemCrypto aims at development and investigation of memristive cryptographic implementations, assessment and improvement of their security against physical attacks. This work focuses on combinational and sequential realizations of complete cryptographic circuits and complements earlier research on memristive physical unclonable functions and random number generators. Simplified cryptographic circuits are physically built out of novel electroforming-free memristive devices fabricated as wire-bonded line arrays using pulsed laser deposition. Physical attacks (side-channel analysis and fault injections) against memristive circuits are studied, with a focus on identifying and characterizing novel attack mechanisms that do not exist in conventional CMOS technology. Attack countermeasures and electrical simulation models and procedures for security analysis are being developed.
Representative publications
2025
- Protected memristive implementations of cryptographic functions. Ziang Chen; Li-Wei Chen; Xianyue Zhao; Kefeng Li; Heidemarie Schmidt; Ilia Polian and Nan Du. To appear in Philosophical Transactions of the Royal Society A (2025).
2024
- Understanding Stochastic Behavior of Self- Rectifying Memristors for Error-Corrected Physical Unclonable Functions. Xianyue Zhao; Jonas Ruchti; Christoph Frisch; Kefeng Li; Ziang Chen; Stephan Menzel; Rainer Waser; Heidemarie Schmidt; Ilia Polian; Michael Pehl and Nan Du. IEEE Transactions on Nanotechnology 23, (2024), pp. 490–499. DOI: https://doi.org/10.1109/TNANO.2024.3413888
2023
- On Side-Channel Analysis of Memristive Cryptographic Circuits. Li-Wei Chen; Ziang Chen; Werner Schindler; Xianyue Zhao; Heidemarie Schmidt; Nan Du and Ilia Polian. IEEE Transactions on Information Forensics and Security 18, (2023), pp. 463–476. DOI: https://doi.org/10.1109/TIFS.2022.3223232
2021
- Low-power emerging memristive designs towards secure hardware systems for applications in internet of things. Nan Du; Heidemarie Schmidt and Ilia Polian. Nano Materials Science 3, (2021), pp. 186–204.
Automated Generation of System-Level Test Programs for Characterization of Parametric Device Properties (GS-IMTR Project P5)
Started in 2020
Partners: Prof. Steffen Becker, Institute of Software Technology, University of Stuttgart, Prof. Stefan Wagner, TU Munich Campus Heilbronn
Supported groupmember: Denis Schwachhofer
Summary: System-Level Test (SLT), where actual application software is run on the circuit, has emerged as an important additional test method for modern Systems-on-Chip (SoCs) that incorporate heterogeneous components, such as multiple processor cores, on-chip memories, application-specific digital circuitry or input-output interfaces. This project focuses on generation of SLT programs with desired characteristics, including extra-functional properties such as power consumption, from high-level software architecture models. It uses a mix of technologies from different domains: fuzzing; genetic programming; and large-language models, and combines simulations with practical experiments.
Representative publications
2024
- Large Language Model-Based Optimization for System-Level Test Program Generation. Denis Schwachhofer; Peter Domanski; Steffen Becker; Stefan Wagner; Matthias Sauer; Dirk Pflüger and Ilia Polian. In 2024 IEEE International Symposium on Defect and Fault Tolerance in VLSI and Nanotechnology Systems (DFT), 2024, pp. 1–6. DOI: https://doi.org/10.1109/DFT63277.2024.10753556
- Optimizing System-Level Test Program Generation via Genetic Programming. Denis Schwachhofer; Francesco Angione; Steffen Becker; Stefan Wagner; Matthias Sauer; Paolo Bernardi and Ilia Polian. In 2024 IEEE European Test Symposium (ETS), 2024, pp. 1–4. DOI: https://doi.org/10.1109/ETS61313.2024.10567817
2023
- Automating Greybox System-Level Test Generation. Denis Schwachhofer; Maik Betka; Steffen Becker; Stefan Wagner; Matthias Sauer and Ilia Polian. In 2023 IEEE European Test Symposium (ETS), Venice, Italy, 2023, pp. 1–4. DOI: https://doi.org/10.1109/ETS56758.2023.10173985
Systematic Analysis of System-Level Test Fails (GS-IMTR Project P1)
Started in 2019
Partner: Prof. Jens Anders, Institute of Smart Sensors, University of Stuttgart
Supported groupmember: Nourhan ElHamawy
Summary: System-level test (SLT) is applied as an additional test insertion to improve test quality, i.e., to prevent defective circuits from passing post-manufacturing tests unnoticed. The objective of this project is to understand the nature of failures that are identified only at the SLT stage, having passed all regular test insertions. Among possible explanations for such SLT-unique fails are complex defect mechanisms not adequately covered by standard fault models; systematic ATPG coverage holes related to, e.g., clock domain boundaries, asynchronous or analog interfaces or clock distribution networks; and marginal defects exposed only during system-level interactions. This project aims at establishing a theoretical and systematic understanding of SLT-unique fails, identifying specific mechanisms leading to such fails and their manifestation conditions, and to explore solutions for their prevention. To this end, we created an experimentation environment where SLT-unique fails can be reproduced and practically investigated.
Representative publications
2025
- Towards understanding of system-level test-unique fails. Nourhan Elhamawy; Jens Anders; Ilia Polian and Matthias Sauer. In IEEE European Test Symp. (ETS), Tallinn, EE, 2025.
2024
- Scenario-based Test Content Optimization: Scan Test vs. System-Level Test. Nourhan Elhamawy; Jens Anders; Ilia Polian and Matthias Sauer. In 2024 IEEE 42nd VLSI Test Symposium (VTS), 2024, pp. 1–7. DOI: https://doi.org/10.1109/VTS60656.2024.10538586
2020
- Exploring the mysteries of system-level test. Ilia Polian; Jens Anders; Stefan Becker; Paolo Bernardi; Krishnendu Chakrabarty; Nourhan Elhamawy; Matthias Sauer; Adith Singh; Matteo Sonza Reorda and Stefan Wagner. In Proceedings of the 29th IEEE Asian Test Symposium (ATS′20), 2020.
Near-Sensor Computing mit ressourceneffizienten Stochastischen Schaltungen (DFG Project)
Started in 2019
Collaborators: Prof. John Hayes, University of Michigan, Ann Arbor, USA, Prof. Stefan Holst and Prof. Kohei Miyase, Kyushu Institute of Technology, Japan
Supported groupmember: Roshwin Sengupta, Florian Neugebauer
Summary: Near-sensor computing is a concept for “sensory swarm” systems, where acquisition of data by sensors is tightly intertwined with computations on these data,.that aims at avoiding the transmission of raw data over relatively slow, power-hungry and potentially unreliable and insecure communication channels. This approach can enable applications like environmental monitoring, surveillance of remote areas and health data tracking by implantable devices, where the sensor nodes are extremely resource-restricted. This project aims at developing methods to realize low-cost and power-efficient hardware circuits for near-sensor computing following the Stochastic Computing paradigm. Stochastic computing provides extremely compact, error-tolerant and low-power implementations of complex functions, but at the expense of longer computation times and some degree of inaccuracy. A special focus of this project is the SC realization of neural networks (NNs) architectures enriched by signal-processing functions such as filters, Fourier and Wavelet transforms.
Representative publications
2025
- WASENN: Wavelet Assisted Stochastic Enabled Neural Network for Human Activity Recognition. Roshwin Sengupta; Ilia Polian and John P. Hayes. IEEE Transactions on Circuits and Systems I: Regular Papers (2025), pp. 1–13. DOI: https://doi.org/10.1109/TCSI.2025.3540564
2024
- Fault tolerance in stochastic circuits for recurrent sequential neural networks. Roshwin Sengupta; Ilia Polian and John P. Hayes. In To appear in Proc. of IEEE Asian Test Symp. (ATS), 2024.
2023
- Stochastic Computing as a Defence Against Adversarial Attacks. Florian Neugebauer; Vivek Vekariya; Ilia Polian and John P. Hayes. In 2023 53rd Annual IEEE/IFIP International Conference on Dependable Systems and Networks Workshops (DSN-W), Porto, Portugal, 2023, pp. 191–194. DOI: https://doi.org/10.1109/DSN-W58399.2023.00053
2022
- On the Impact of Hardware Timing Errors on Stochastic Computing based Neural Networks. Florian Neugebauer; Stefan Holst and Ilia Polian. In 2022 IEEE European Test Symposium (ETS), 2022, pp. 1–6. DOI: https://doi.org/10.1109/ETS54262.2022.9810429
2020
- Hardware-based fast real-time image classification with stochastic computing. Ponana Kelettira Muthappa; Florian Neugebauer; Ilia Polian and John P. Hayes. In Proceedings of the 38th IEEE International Conference on Computer Design (ICCD′20), 2020.
Contact
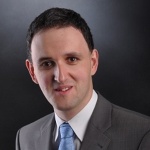
Ilia Polian
Prof. Dr. rer. nat. habil.Head of Institute and Chair of Hardware Oriented Computer Science